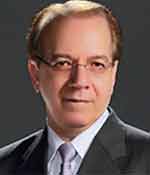
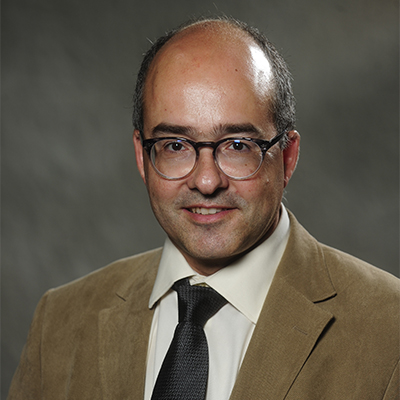
Emphasis
The emphasis is on development of transformative machine intelligence-based systems, emerging tools, and modern technologies for diagnosing and recommending treatments for a range of diseases and health conditions. Unsupervised and semi-supervised techniques and methodologies are of particular interest.
Program priorities and areas of interest:
- clinical decision support systems
- computer-aided diagnosis
- computer-aided screening
- analyzing complex patterns and images
- screening for diseases
- natural-language processing and understanding
- medical decision-making
- predictive modeling
- computer vision
- robotic and image guided surgery
- personalized imaging and treatment
- drug discovery
- radiomics
- machine/deep learning-based segmentation, registration, etc.
Additional support
This program also supports:
- early-stage development of software, tools, and reusable convolutional neural networks
- data reduction, denoising, improving performance (health-promoting apps), and deep-learning based direct image reconstruction
- approaches that facilitate interoperability among annotations used in image training databases
Related News
The National Institutes of Health (NIH) has selected four finalists with innovative, non-invasive technologies that seek to improve diagnosis of endometriosis.
The low image quality of small, affordable MRI machines have prevented their widespread use. But a boost from AI could close the gap, bringing MRI to more patients.
NIH has awarded more than $4 million in funds and support services to three diagnostic technology developers as part of RADx ® Tech’s Advanced Platforms for HIV Viral Load Monitoring program.
A tiny, four-fingered “hand” folded from a single piece of DNA can pick up the virus that causes COVID-19 for highly sensitive rapid detection and can even block viral particles from entering cells to infect them, University of Illinois Urbana-Champaign researchers report. Source: University of Illinois Urbana-Champaign News Bureau
As AI is deployed in clinical centers across the U.S., one important consideration is to assure that models are fair and perform equally across patient groups and populations. To better understand the fairness of medical imaging AI, researchers trained over 3,000 models spanning multiple model configurations, algorithms, and clinical tasks. Their analysis of these models reinforced some previous findings about bias in AI algorithms and uncovered new insights about deployment of models in diverse settings.