Of all the instruments in the modern medicine toolbox, magnetic resonance imaging, or MRI, is one of the most powerful. But in many regions, the expense, space, and expertise required to use it puts this tool out of reach. A new study suggests that, with a performance boost from artificial intelligence (AI), existing portable, low-cost MRI tech could make high-quality brain imaging available to more patients.
“The idea is to bring the scanner to the patient. It could be applied very broadly, in the ICU, or out into communities. The potential applications are very broad,” said corresponding author W. Taylor Kimberly, M.D., Ph.D., a critical care neurologist at Massachusetts General Hospital and associate professor of neurology at Harvard Medical School.
The new system employs AI techniques to improve the quality of, and then automatically analyze, images taken from low-field strength MRI devices that, due to being smaller, sacrifice image quality compared to conventional MRI machines. In a recent clinical study, researchers from Massachusetts General Hospital, Harvard Medical School, and Yale School of Medicine used their automated approach to detect Alzheimer’s disease markers in humans, finding that their technique performed at the same level as higher-power MRI. Their results, published in Nature Communications, point to the new method as a potential means of providing broad access to high-quality brain imaging.
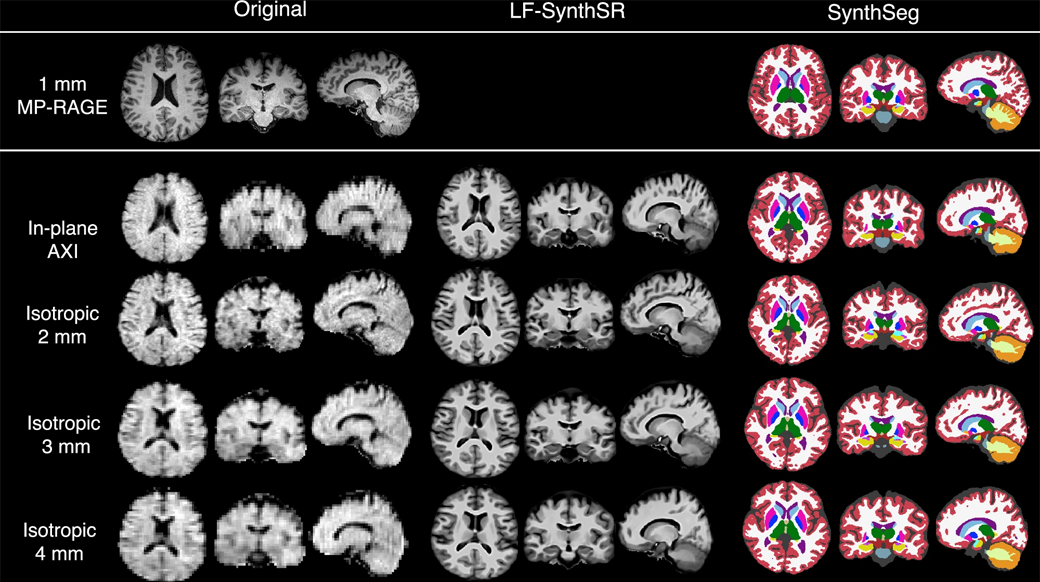
“Researchers have dreamed of scaling down MRI without reducing its usefulness, but it’s technologically difficult. By really pressing their analytical capabilities with AI, this team has brought that dream of affordable imaging much closer to reality,” said Guoying Liu, Ph.D., director of the Division of Applied Science and Technology at the National Institute of Biomedical Imaging and Bioengineering (NIBIB).
Early diagnosis and monitoring for dementia is critical, and with the recent emergence of early interventions for the condition, it may only become more important. To gain insights into dementia, clinicians prefer to employ MRI when they can, but since it’s often not practical, patients frequently go undiagnosed. For years, researchers have been investigating low-field strength MRI devices as an alternative.
Like traditional MRI, low-field MRI works in part by exposing tissues to magnetic fields. Their magnets are just smaller, producing fields that are tens to hundreds of times weaker than their high-field counterparts. These lightweight devices take up less space than standard machines – which often have entire rooms dedicated to them – but the tradeoff is usually a steep drop off in image quality, Kimberly explained.
Not only may images be too fuzzy for human eyes to discern important details, but their lack of clarity could also rule out fully or semi-automated image analysis. Many of these timesaving tools require images with voxels (like pixels, but in 3D) that are at most 1 millimeter in size.
Kimberly together with a multidisciplinary team of clinicians and biomedical engineers set out to enhance the capabilities of low-field tech. A key piece of their game plan was to improve the resolution of images through machine learning.
“Our brains are all unique, of course, but there are many, many common features across individuals. This is why machine learning is useful here, because an algorithm can learn those features and then fill in the gaps in a lower resolution image,” Kimberly said.
An algorithm’s performance is only as good as its training allows and in an arena such as brain imaging, there is not much room for error. To create a system that would be resilient against a wide range of variability, the team trained algorithms on brain scans representing a range of contexts.
In a previous study, the team created an AI model that could boost low-quality scans taken using a portable, low-field MRI machine to the crucial 1-millimeter resolution. The researchers found that the generated high-resolution images had good agreement with actual high-resolution images taken from a small group of patients.
On top of increasing the quality of images acquired by low-field MRI, the researchers also developed fully automated programs to identify different regions of the brain and then calculate the volume of parts of interest. In the new work, the authors cranked up the difficulty by deploying low-field MRI and their machine learning tools in a larger number of people, including many patients with Alzheimer’s disease or mild cognitive impairment (MCI).
In particular, they used their automated approach to calculate the size of the hippocampus and lateral ventricle – regions of the brain that are often altered in Alzheimer’s disease. They also used the system to identify and measure lesions in the brain, called white matter hyperintensities, also linked to Alzheimer’s. Tracking changes in these regions is useful for both diagnosing and monitoring the progression of degenerative conditions.
Throughout experiments across nearly 100 subjects, the results from their AI-enhanced, low-field MRI system were on par with measurements taken with conventional, high-field machines. The authors showed that both methods could distinguish the brains of subjects with Alzheimer’s disease or MCI from those that were healthy at a similar level of accuracy.
“Time and time again, in all of the contexts that we’ve used these algorithms, they have demonstrated to be very robust,” Kimberly said.
The researchers believe that with some further refinement, the method could give many more patients a chance to have their brain health assessed via high-quality brain imaging, a resource that may only become more critical in the future.
This study was supported in part by grants from NIBIB (R01EB031114), the National Institute of Mental Health (RF1MH123195 and UM1MH130981), and the National Institute on Aging (R01AG07098 and RF1AG080371).
Study author Matthew S. Rosen, Ph.D., is an equity holder of Hyperfine, Inc., which produced the low-field MRI scanners used in this study.
About the graphic: The image, which originally appeared in Nature Communications, is available under the Creative Commons license, and has been adapted for the NIBIB website.
Study reference: Annabel J. Sorby-Adams et al. Portable, low-field magnetic resonance imaging for evaluation of Alzheimer’s disease. Nature Communications (2024). https://doi.org/10.1038/s41467-024-54972-x