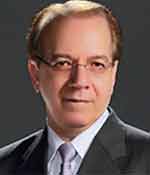
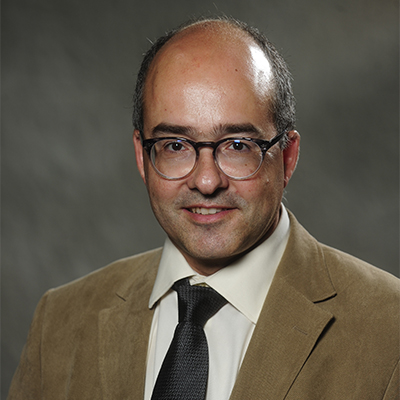
Emphasis
The emphasis is on development of transformative machine intelligence-based systems, emerging tools, and modern technologies for diagnosing and recommending treatments for a range of diseases and health conditions. Unsupervised and semi-supervised techniques and methodologies are of particular interest.
Program priorities and areas of interest:
- clinical decision support systems
- computer-aided diagnosis
- computer-aided screening
- analyzing complex patterns and images
- screening for diseases
- natural-language processing and understanding
- medical decision-making
- predictive modeling
- computer vision
- robotic and image guided surgery
- personalized imaging and treatment
- drug discovery
- radiomics
- machine/deep learning-based segmentation, registration, etc.
Additional support
This program also supports:
- early-stage development of software, tools, and reusable convolutional neural networks
- data reduction, denoising, improving performance (health-promoting apps), and deep-learning based direct image reconstruction
- approaches that facilitate interoperability among annotations used in image training databases
Related News
Tiny implantable sensors are helping University of Oregon researchers optimize the process of recovery from severe bone injuries. In a new study, they use the technology to show that a resistance-training rehabilitation program can significantly improve femur injuries in rats in just eight weeks.
Source: University of Oregon Phil and Penny Knight Campus for Accelerating Scientific Impact
Five NIBIB grantees received the Presidential Early Career Award for Scientists and Engineers: Ambika Goel Bajpayee, Northeastern University; Samira Kiani, University of Pittsburgh; Kanaka Rajan, Harvard Medical School; Parisa Rashidi, University of Florida; and William Renninger, University of Rochester. The award is the highest honor bestowed by the U.S. Government on outstanding scientists and engineers at the early career stage. White House Announcement
In an effort to greatly expand accessibility, this compact fluorescence-guided surgery system, evaluated in mice, is crafted from cost-effective and off-the-shelf components.
AI, specifically, machine learning, can help improve the quality of these MRI images, making it easier for doctors to diagnose patients. Machine learning involves training AI on a lot of data to recognize trends and make predictions on new data it receives. Source: Curious Science Writers
Rice University bioengineers have developed a new construction kit for building custom sense-and-respond circuits in human cells. The research, published in the journal Science, represents a major breakthrough in the field of synthetic biology that could revolutionize therapies for complex conditions like autoimmune disease and cancer. Source: Rice University News & Media Relations